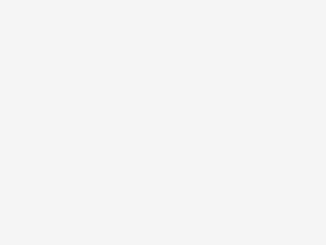
The Python Language Summit 2023: Pattern Matching, __match__, and View Patterns
How does working with ASTs relate to pattern-matching? Well, a function to determine whether (to a reasonable approximation) an arbitrary AST node represents the symbol collections.deque
might have looked something like this, before pattern matching…
import ast
# This obviously won't work if the symbol is imported with an alias
# in the source code we're inspecting
# (e.g. "from collections import deque as d").
# But let's not worry about that here :-)
def node_represents_collections_dot_deque(node: ast.AST) -> bool:
"""Determine if *node* represents 'deque' or 'collections.deque'"""
return (
isinstance(node, ast.Name) and node.id == "deque"
) or (
isinstance(node, ast.Attribute)
and isinstance(node.value, ast.Name)
and node.value.id == "collections"
and node.value.attr == "deque"
)
But in Python 3.10, pattern matching allows an elegant destructuring syntax:
import ast
def node_represents_collections_dot_deque(node: ast.AST) -> bool:
"""Determine if *node* represents 'deque' or 'collections.deque'"""
match node:
case ast.Name("deque"):
return True
case ast.Attribute(ast.Name("collections"), "deque"):
return True
case _:
return False
I know which one I prefer.
For some, though, this still isn’t enough – and Michael “Sully” Sullivan is one of them. At the Python Language Summit 2023, Sullivan shared ideas for where pattern matching could go next.
Playing with matches (without getting burned)
Sullivan’s contention is that, while pattern matching provides elegant syntactic sugar in simple cases such as the one above, our ability to chain destructurings using pattern matching is currently fairly limited. For example, say we want to write a function inspecting Python AST that takes an ast.FunctionDef
node and identifies whether the node represents a synchronous function with exactly two parameters, both of them annotated as accepting integers. The function would behave so that the following holds true:
>>> import ast
>>> source = "def add_2(number1: int, number2: int): pass"
>>> node = ast.parse(source).body[0]
>>> type(node)
<class 'ast.FunctionDef'>
>>> is_function_taking_two_ints(node)
True
With pre-pattern-matching syntax, we might have written such a function like this:
def is_int(node: ast.AST | None) -> bool:
"""Determine if *node* represents 'int' or 'builtins.int'"""
return (
isinstance(node, ast.Name) and node.id == "int"
) or (
isinstance(node, ast.Attribute)
and isinstance(node.value, ast.Name)
and node.value.id == "builtins"
and node.attr == "int"
)
def is_function_taking_two_ints(node: ast.FunctionDef) -> bool:
"""Determine if *node* represents a function that accepts two ints"""
args = node.args.posonlyargs + node.args.args
return len(args) == 2 and all(is_int(node.annotation) for node in args)
def is_int(node: ast.AST | None) -> bool:
"""Determine if *node* represents 'int' or 'builtins.int'"""
match node:
case ast.Name("int"):
return True
case ast.Attribute(ast.Name("builtins"), "int"):
return True
case _:
return False
def is_function_taking_two_ints(node: ast.FunctionDef) -> bool:
"""Determine if *node* represents a function that accepts two ints"""
match node.args.posonlyargs + node.args.args:
case [ast.arg(), ast.arg()] as arglist:
return all(is_int(arg.annotation) for arg in arglist)
case _:
return False
That leaves a lot to be desired, however! The is_int()
helper function can be rewritten in a much cleaner way. But integrating it into the is_function_taking_two_ints()
is… somewhat icky! The code feels harder to understand than before, whereas the goal of pattern matching is to improve readability.
Something like this, (ab)using metaclasses, gets us a lot closer to what it feels pattern matching should be like. By using one of Python’s hooks for customising isinstance()
logic, it’s possible to rewrite our is_int()
helper function as a class, meaning we can seamlessly integrate it into our is_function_taking_two_ints()
function in a very expressive way:
import abc
import ast
class PatternMeta(abc.ABCMeta):
def __instancecheck__(cls, inst: object) -> bool:
return cls.match(inst)
class Pattern(metaclass=PatternMeta):
"""Abstract base class for types representing 'abstract patterns'"""
@staticmethod
@abc.abstractmethod
def match(node) -> bool:
"""Subclasses must override this method"""
raise NotImplementedError
class int_node(Pattern):
"""Class representing AST patterns signifying `int` or `builtins.int`"""
@staticmethod
def match(node) -> bool:
match node:
case ast.Name("int"):
return True
case ast.Attribute(ast.Name("builtins"), "int"):
return True
case _:
return False
def is_function_taking_two_ints(node: ast.FunctionDef) -> bool:
"""Determine if *node* represents a function that accepts two ints"""
match node.args.posonlyargs + node.args.args:
case [
ast.arg(annotation=int_node()),
ast.arg(annotation=int_node()),
]:
return True
case _:
return False
This is still hardly ideal, however – that’s a lot of boilerplate we’ve had to introduce to our helper function for identifying int
annotations! And who wants to muck about with metaclasses?
A slide from Sullivan’s talk |
A __match__
made in heaven?
Sullivan proposes that we make it easier to write helper functions for pattern matching, such as the example above, without having to resort to custom metaclasses. Two competing approaches were brought for discussion.
The first idea – a __match__
special method – is perhaps the easier of the two to immediately grasp, and appeared in early drafts of the pattern matching PEPs. (It was eventually removed from the PEPs in order to reduce the scope of the proposed changes to Python.) The proposal is that any class could define a __match__
method that could be used to customise how match statements apply to the class. Our is_function_taking_two_ints()
case could be rewritten like so:
class int_node:
"""Class representing AST patterns signifying `int` or `builtins.int`"""
# The __match__ method is understood by Python to be a static method,
# even without the @staticmethod decorator,
# similar to __new__ and __init_subclass__
def __match__(node) -> ast.Name | ast.Attribute:
match node:
case ast.Name("int"):
# Successful matches can return custom objects,
# that can be bound to new variables by the caller
return node
case ast.Attribute(ast.Name("builtins"), "int"):
return node
case _:
# Return `None` to indicate that there was no match
return None
def is_function_taking_two_ints(node: ast.FunctionDef) -> bool:
"""Determine if *node* represents a function that accepts two ints"""
match node.args.posonlyargs + node.args.args:
case [
ast.arg(annotation=int_node()),
ast.arg(annotation=int_node()),
]:
return True
case _:
return False
The second idea is more radical: the introduction of some kind of new syntax (perhaps reusing Python’s ->
operator) that would allow Python coders to “apply” functions during pattern matching. With this proposal, we could rewrite is_function_taking_two_ints()
like so:
def is_int(node: ast.AST | None) -> bool:
"""Determine if *node* represents 'int' or 'builtins.int'"""
match node:
case ast.Name("int"):
return True
case ast.Attribute(ast.Name("builtins"), "int"):
return True
case _:
return False
def is_function_taking_two_ints(node: ast.FunctionDef) -> bool:
"""Determine if *node* represents a function that accepts two ints"""
match node.args.posonlyargs + node.args.args:
case [
ast.arg(annotation=is_int -> True),
ast.arg(annotation=is_int -> True),
]
case _:
return False
Match-maker, match-maker, make me a __match__
…
The reception in the room to Sullivan’s ideas was positive; the consensus seemed to be that there was clearly room for improvement in this area. Brandt Bucher, author of the original pattern matching implementation in Python 3.10, concurred that this kind of enhancement was needed. Łukasz Langa, meanwhile, said he’d received many queries from users of other programming languages such as C#, asking how to tackle this kind of problem.
The proposal for a __match__
special method follows a pattern common in Python’s data model, where double-underscore “dunder” methods are overridden to provide a class with special behaviour. As such, it will likely be less jarring, at first glance, to those new to the idea. Attendees of Sullivan’s talk seemed, broadly, to slightly prefer the __match__
proposal, and Sullivan himself said he thought it “looked prettier”.
Jelle Zijlstra argued that the __match__
dunder would provide an elegant symmetry between the construction and destruction of objects. Brandt Bucher, meanwhile, said he thought the usability improvements weren’t significant enough to merit new syntax.
Nonetheless, the alternative proposal for new syntax also has much to recommend it. Sullivan argued that having dedicated syntax to express the idea of “applying” a function during pattern matching was more explicit. Mark Shannon agreed, noting the similarity between this idea and features in the Haskell programming language. “This is functional programming,” Shannon argued. “It feels weird to apply OOP models to this.”
Addendum: pattern-matching resources and recipes
In the meantime, while we wait for a PEP, there are plenty of innovative uses of pattern matching springing up in the ecosystem. For further reading/watching/listening, I recommend:
- “A perfect
match
: The history, design and future of Python’s structural pattern matching” – A talk by Brandt Bucher at PyCon 2022 - “Structural Pattern Matching in the Real World” – A talk by Raymond Hettinger at Pycon Italia 2022
RegexMatcher
: a class integrating pattern matching with Python’sre
module. A 2022 Advent of Code solution by Ned Batchelder.approximately
: A way to comparefloat
andcomplex
numbers using pattern matching, while avoiding the perils of floating-point arithmetic. A StackOverflow Q&A by Raymond Hettinger.- “A few related schemes for implementing view patterns in Python”: A gist by Michael Sullivan (from February 2023)
That’s a lot of words which may or may not mean very much to you – but consider, for example, using the
ast
module to parse Python source code. If you’re unfamiliar with theast
module: the module provides tools that enable you to compile Python source code into an “abstract syntax tree” (AST) representing the code’s structure. The Python interpreter itself converts Python source code into an AST in order to understand how to run that code – but parsing Python source code using ASTs is also a common task for linters, such as plugins for flake8 or pylint. In the following example,ast.parse()
is used to parse the source codex = 42
into anast.Module
node, andast.dump()
is then used to reveal the tree-like structure of that node in a human-readable form: